Content
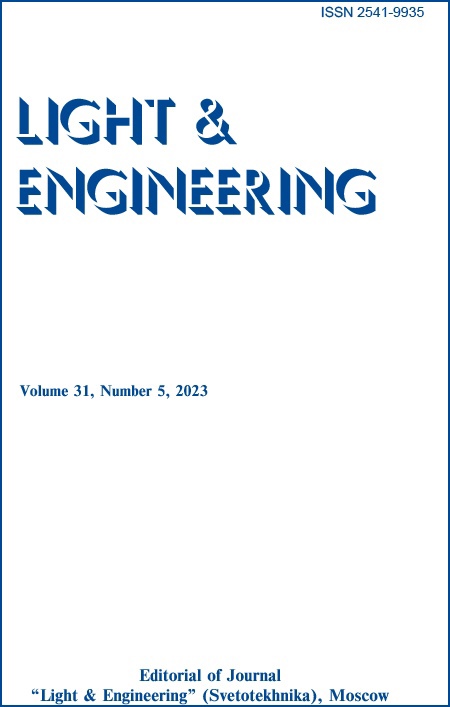
Light & Engineering 31 (5)
Volume 31Date of publication 10/10/2023
Pages 135–142
Abstract:
Convolution neural networks are widely used for image processing in remote sensing. Aquacultures have an important role in food security and hence should be monitored. In this paper, a novel lightweight neural network for in-terrestrial aquaculture field retrieval from high-resolution remote sensing images is proposed. The structure of this pond segmentation network is based on the UNet architecture, providing higher training speed. Experiments are performed on Gaofen satellite datasets in Shanghai, China. The proposed network detects the inland aquaculture ponds in a shorter time than stateof-the-art neural network-based models and reaches an overall accuracy of about 90 %.
References:
1. Liang, C., Cheng, B., Xiao, B., He, C., Liu, X., Jia, N., Chen, J. Semi-/Weakly-Supervised Semantic Segmentation Method and Its Application for Coastal Aquaculture Areas Based on Multi-SourceRemote Sensing Images // Taking the Fujian Coastal Area (Mainly Sanduo) as an Example. Remote Sens, 2021, # 13, 1083 p. 2. Yu, C., Liu, Y., Hu, Z., Xia, X. Accurate segmentation of remote sensing cages based on U-Net and voting mechanism // Proc. SPIE12166, Seventh Asia Pacific Conference on Optics Manufacture and 2021 International Forum of Young Scientists on Advanced Optical Manufacturing (APCOM and YSAOM 2021), 2022, # 121662T. 3. Zhu, H., Li, K., Wang, L., Chu, J., Gao, N., Chen, Y. Spectral Characteristic Analysis and Remote Sensing Classification of Coastal Aquaculture Areas Based on GF‑1 Data // Journal of Coastal Research, 2019, Vol. 90, # sp1, pp. 49–57. 4. Zhu, X.X. et al. Deep Learning in Remote Sensing: A Comprehensive Review and List of Resources // in IEEE Geoscience and Remote Sensing Magazine, 2017, Vol. 5, # 4, pp. 8–36. 5. Zou, Z., Chen, C., Liu, Z., Zhang, Z., Liang, J., Chen, H., Wang, L. Extraction of Aquaculture Ponds along Coastal Region Using U2-Net Deep Learning Model from Remote Sensing Images // Remote Sensing, 2022, Vol. 14, # 16, 4001 p. 6. Lu, Y., Shao, W., Sun, J. Extraction of Offshore Aquaculture Areas from Medium-Resolution Remote Sensing Images Based on Deep Learning // Remote Sensing, 2021, Vol. 13, # 19, 3854 p. 7. LeCun, Y., Bengio, Y., Hinton, G. Deep learning // Nature, 2015, Vol. 521, pp. 436–444. 8. Kussul, N., Lavreniuk, M., Skakun, S., Shelestov, A. Deep Learning Classification of Land Cover and Crop Types Using Remote Sensing Data // in IEEE Geoscience and Remote Sensing Letters, 2017, Vol. 14, # 5, pp. 778–782. 9. Abebaw, A., Shailender, K. Transfer Learning Models for Land Cover and Land Use Classification in Remote Sensing Image // Applied Artificial Intelligence, 2022, Vol. 36, # 1. 10. Mohammadi, M., Sharifi, A. Evaluation of Convolutional Neural Networks for Urban Mapping Using Satellite Images // J Indian Soc Remote Sens, 2021, Vol. 49, pp. 2125–2131. 11. Bell, T.W., Nidzieko, N.J., Siegel, D.A., Miller, R.J., Cavanaugh, K.C., Nelson, N.B., Reed, D.C., Fedorov, D., Moran, C., Snyder, J.N., Cavanaugh, K.C., Yorke, C.E., Griffith, M. The Utility of Satellites and Autonomous Remote Sensing Platforms for Monitoring Offshore Aquaculture Farms: A Case Study for Canopy Forming Kelps // Front. Mar. Sci., 2020, Vol. 7, # 520223. 12. Abdollahi, A., Pradhan, B., Shukla, N., Chakraborty, S., Alamri, ADeep Learning Approaches Applied to Remote Sensing Datasets for Road Extraction: A State-Of-The-Art Review // Remote Sensing, 2020, Vol. 12, # 9, 1444 p. 13. Chen, K., Fu, K., Gao, X., Yan, M., Sun, X., Zhang, H. Building extraction from remote sensing images with deep learning in a supervised manner // 2017 IEEE International Geoscience and Remote Sensing Symposium (IGARSS), 2017. 14. Al Mansoori, S., Kunhu, A., AlHammadi, A. Effective Airplane Detection in High Resolution Satellite Images using YOLOv3 Model // 2021 4th International Conference on Signal Processing and Information Security (ICSPIS), 2021, pp. 57–60. 15. Huete, A.R. Remote Sensing for Environmental Monitoring Environmental Monitoring and Characterization // Elsevier, 2004, pp. 183–206. 16. Sandler, M., Howard, A., Zhu, M., Zhmoginov, A., Chen, L.-C. MobileNetV2: Inverted Residuals and Linear Bottlenecks // 2018 IEEE/CVF Conference on Computer Vision and Pattern Recognition, 2018, pp. 4510–4520. 17. Badrinarayanan, V., Kendall, A., Cipolla, R. Seg-Net: A Deep Convolutional Encoder-Decoder Architecture for Image Segmentation // IEEE Transactions on Pattern Analysis and Machine Intelligence, 2017, Vol. 39, # 12, pp. 2481–2495. 18. Gao, H., Gu, X., Yu, T., Liu, L., Sun, Y., Xie, Y., Liu, Q. Validation of the Calibration Coefficient of the GaoFen‑1 PMS Sensor Using the Landsat 8 OLI // Remote Sensing, 2016, Vol. 8, # 2, 132 p. 19. Liu, Y., Li, C., Ma, L., Wang, N., Qian, Y., Tang, L. Vicarious absolute radiometric calibration of GF‑2 PMS2 sensor using permanent artificial targets in China // Proc. SPIE10000, Sensors, Systems, and Next-Generation Satellites XX, 2016, # 1000019. 20. Korkmaz, S.A., Akcicek, A., Binol, H., Korkmaz, Mehmet, F. Recognition of the stomach cancer images with probabilistic HOG feature vector histograms by using HOG features // 2017 IEEE15th International Symposium on Intelligent Systems and Informatics (SISY), 2017, pp. 000339–000342. 21. Ronneberger, O., Fischer, P., Brox, T. U-Net: Convolutional Networks for Biomedical Image Segmentation, 2015, # May 2015. 22. Ronneberger, O., Fischer, P., Brox, T. U-Net: Convolutional Networks for Biomedical Image Segmentation. In: Navab, N., Hornegger, J., Wells, W., Frangi, A. (eds) Medical Image Computing and Computer-Assisted Intervention – MICCAI 2015, MICCAI 2015 / Lecture Notes in Computer Science, 2015, Vol. 9351. 23. Su, H., Wei, S., Qiu, J., Wu, W. RaftNet: A New Deep Neural Network for Coastal Raft Aquaculture Extraction from Landsat 8 OLI Data // Remote Sens, 2022, Vol. 14, # 4587. 24. Kataev M. Yu., Dadonova M.M. Method of Vegetation Detection Using RGB Images Made by Unmanned Aerial Vehicles on the Basis of Colour and Texture Analysis // Light & Engineering, 2019, Vol. 27, # 5, pp. 55–62. 25. Chen, T. Wu, Q.H., Rahmani-Torkaman, R., Hughes, J. A pseudo top-hat mathematical morphological approach to edge detection in dark regions // The Journal of the Pattern Recognition Society, 2002, Vol. 35, #1, pp. 199–210. 26. Zhang, J., Feng, M.Q., Wang, Y. Automatic segmentation of remote sensing images on water bodies based on image enhancement // Traitement du Signal, 2020, Vol. 37, # 6, pp. 1037–1043.
Keywords
Recommended articles
Discrete Ordinate Radiative Transfer Model With the Neural Network Based Eigenvalue Solver: proof Of Concept Light & Engineering Vol. 29, No. 1
Accuracy Enhancement of the Two-Stream Radiative Transfer Model for Computing Absorption Bands at the Presence of Aerosols L&E, Vol. 29, No. 2, 2021
Application Of The Photometric Theory Of The Radiance Field In The Problems Of Electron Scattering. L&E 27 (2) 2019