Content
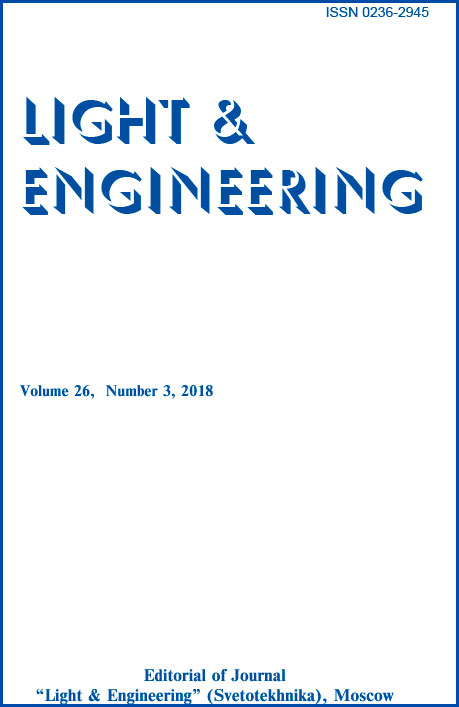
Abstract
BP neural network is a new computer technology, which has natural advantages in the study of lighting environment evaluation model of subway station space. With the implementation of energy conservation and emission reduction, how to establish a suitable calculation model for the lighting environment of subway station space is also a hot research field. Firstly, the development trend of subway and the research status at home and abroad is introduced, then the specific application method of BP neural network is expounded, the evaluation criteria and evaluation model of space lighting environment of subway station is established. BP neural network is used to establish the corresponding mathematical model, through the weight calculation, the appropriate evaluation system is established, and finally the model is used to verify it.
References
1. Englund S R, O’brien J J, Clark D B. Evaluation of digital and film hemispherical photography and spherical densiometry for measuring forest light environments. Canadian Journal of Forest Research, 2000. V30, #12, pp.1999–2005. 2. Poorter L, Arets E J M M. Light environment and tree strategies in a Bolivian tropical moist forest: an evaluation of the light partitioning hypothesis. Plant Ecology, 2003. V166, #2, pp.295–306. 3. Hernandez Duran T, Ravela N, Sanchez Rivero S, et al. Evaluation of Different Light Conditions in the Working Environment for Handling Photosensitive and Thermolabile Compounds. Journal of AOAC International, 2015. V98, #6, pp.1491–1495. 4. Mills P R, Tomkins S C, Schlangen L J M. The effect of high correlated colour temperature office lighting on employee wellbeing and work performance. Journal of circadian rhythms, 2007. V5, #1, p.2. 5. Haiying Li, Xian Li, Xinyue Xu, Jun Liu, Bin Ran. Modeling departure time choice of metro passengers with a smart corrected mixed logit model – A case study in Beijing. Transport Policy. 2018, #69, pp.106–121. 6. Li D H W, Lam J C. Evaluation of lighting performance in office buildings with daylighting controls. Energy and buildings, 2001. V33, #8, pp.793–803. 7. Iwata T, Tokura M, Shukuya M, et al. A pilot experiment on a method for evaluating acceptability of a daylit luminous environment. Journal of Thermal Biology, 1993. V18, #5–6, pp.555–559. 8. Xu, X., Xie, L., Li, H., & Qin, L. Learning the route choice behavior of subway passengers from AFC data. Expert Systems with Applications, 2018. #95, pp. 324–332. 9. Stansbury J, Mittelsdorf A M. Economic and environmental analysis of retrofitting a large office building with energyefficient lighting systems. Environmental management, 2001. V27, #6, pp.909–918. 10. Aghemo C, Fabbri M, Verso V L, et al. Visual perception in aircraft cockpit under real ambient lighting conditions: A procedure for the evaluation of readability and legibility. Perception ECVP abstract, 2009. V38, pp.181–181.
Keywords
Recommended articles
Optimization of Intelligent Illumination in University Classroom Based on FMRAS Control Algorithm . L&E 26 (2) 2018